Full Paper View Go Back
Neural Network Architecture for Signal Processing Analog VLSI Implementation
Priyanjali Jain1 , Priyanshu Jain2
Section:Research Paper, Product Type: Journal-Paper
Vol.7 ,
Issue.4 , pp.63-69, Dec-2020
Online published on Dec 31, 2020
Copyright © Priyanjali Jain, Priyanshu Jain . This is an open access article distributed under the Creative Commons Attribution License, which permits unrestricted use, distribution, and reproduction in any medium, provided the original work is properly cited.
View this paper at Google Scholar | DPI Digital Library
How to Cite this Paper
- IEEE Citation
- MLA Citation
- APA Citation
- BibTex Citation
- RIS Citation
IEEE Style Citation: Priyanjali Jain, Priyanshu Jain, “Neural Network Architecture for Signal Processing Analog VLSI Implementation,” World Academics Journal of Engineering Sciences, Vol.7, Issue.4, pp.63-69, 2020.
MLA Style Citation: Priyanjali Jain, Priyanshu Jain "Neural Network Architecture for Signal Processing Analog VLSI Implementation." World Academics Journal of Engineering Sciences 7.4 (2020): 63-69.
APA Style Citation: Priyanjali Jain, Priyanshu Jain, (2020). Neural Network Architecture for Signal Processing Analog VLSI Implementation. World Academics Journal of Engineering Sciences, 7(4), 63-69.
BibTex Style Citation:
@article{Jain_2020,
author = {Priyanjali Jain, Priyanshu Jain},
title = {Neural Network Architecture for Signal Processing Analog VLSI Implementation},
journal = {World Academics Journal of Engineering Sciences},
issue_date = {12 2020},
volume = {7},
Issue = {4},
month = {12},
year = {2020},
issn = {2347-2693},
pages = {63-69},
url = {https://www.isroset.org/journal/WAJES/full_paper_view.php?paper_id=2236},
publisher = {IJCSE, Indore, INDIA},
}
RIS Style Citation:
TY - JOUR
UR - https://www.isroset.org/journal/WAJES/full_paper_view.php?paper_id=2236
TI - Neural Network Architecture for Signal Processing Analog VLSI Implementation
T2 - World Academics Journal of Engineering Sciences
AU - Priyanjali Jain, Priyanshu Jain
PY - 2020
DA - 2020/12/31
PB - IJCSE, Indore, INDIA
SP - 63-69
IS - 4
VL - 7
SN - 2347-2693
ER -
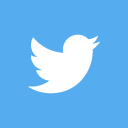
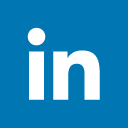
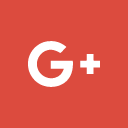
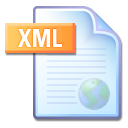
Abstract :
With the advent of new technologies and advancement in medical science we are trying to process the information artificially as our biological system performs inside our body. Artificial intelligence through a biological word is realized based on mathematical equations and artificial neurons. Our main focus is on the implementation of Neural Network Architecture (NNA) with on a chip learning in analog VLSI for generic signal processing applications. In the proposed paper analog components like Gilbert Cell Multiplier (GCM), Neuron activation Function (NAF) are used to implement artificial NNA. The analog components used are comprises of multipliers and adders’ along with the tan-sigmoid function circuit using MOS transistor in subthreshold region. This neural architecture is trained using Back propagation (BP) algorithm in analog domain with new techniques of weight storage. Layout design and verification of the proposed design is carried out using Tanner EDA 14.1 tool and synopsys Tspice. The technology used in designing the layouts is MOSIS/HP 0.5u SCN3M, Tight Metal.
Key-Words / Index Term :
Back Propagation, Algorith, Neural Network Architecture
References :
[1] Cyril Prasanna Raj P & S.L. Pinnae “DESIGN AND ANALOG VLSI IMPLEMENTATION OF NEURAL NETWORK ARCHITECTURE FOR SIGNAL PROCESSING” European Journal of Scientific Research ISSN 1450-216X Vol.27No.2 (2009), pp.199-216
[2] George Papadourakis “INTRODUCTION TO NEURAL NETWORKS” (2009)
[3] Anita Wasilewska “NEURAL NETWORKS” State University of New York at Stony Brook.
[4] Ranjeet Ranade & Sanjay Bhandari & A.N. Chandorkar “VLSI Implementation of Artificial Neural Digital Multiplier and Adder” pp.318-319
[5] Rafid Ahmed Khalil & Sa`ad Ahmed Al-Kazzaz “Digital Hardware Implementation of Artificial Neurons Models Using FPGA”pp.12-24
[6] Bose N.K., Liang P., Neural Network Fundamentals With graphs, algorithms and Application, Tata McGraw hill, New Delhi, 2002,ISBN0-07-463529-8.
[7] Razavi Behzad, Design of Analog CMOS Integrated Circuits, Tata McGraw hill, New Delhi , 2002, ISBN0-07-052903-5.
[8] Roy Ludvig Sigvartsen, An Analog Neural Network With On-Chip Learning Thesis Department of informatics, University of Oslo, 1994
[9] Isik Aybayetal , Classification of Neural Network Hardware, Neural Network World ,IDG Co., Vol6 No1, 1996, pp.11-29 ,
[10] Vincent F. Koosh Analog Computation and Learning in VLSI PhD thesis California institute of technology, Pasadena, California.2001.
[11] E. Vittoz, et al.,” The design of high performance analog circuits on digital CMOS chips,” IEEE J. Solid State Circuit 20, 1985, pp.657-665
[12] S. Orcioni G. Biagetti, M. Conti, “A mixed signal fuzzy controller using current model circuits, “ Analog Integrated Circuits Process. 38, 2004 , pp.215-231.
[13] F. Djeffal et al., “Design and Simulation of Nanoelectronic DG MOSFET Current Source using Artificial Neural Networks,” Materials Science and Engineering C, vol. 27, 2007, pp. 1111-1116
[14] H. Ben Hommounda et al., “Neural Based Models Of Semiconductor Devices for Spice Simulator,”American J. of Applied Science, vol. 5, 2008, pp. 385-391
[15] Arne Heittmann, “An Analog VLSI Pulsed Neural Network for Image Segmentation using Adaptive Connection Weights” Dresden University of Technology, Department of Electrical Engineering and Information Technology,Dresden, Germany, 2000
[16] Wai-Chi Fang et al, “A VLSI Neural Processor for Image Data Compression using Self-Organisation Networks” IEEE Transactions on Neural Networks, Vol. 3, No. 3, May 1992, pp. 506-517
[17] R. Rojas, Neural Networks, Springer-Verlag, Berlin, 1996
[18] D. Nguyen a and B. Wid row, Improving the learning speed of 2-layer neural network by choosing initial values of the adaptive weights, IEEE First International Joint Conference on Neural Networks , 3, 21–26, (1990).
[19] R.A. Jacobs, Increased rates of convergence through learning rate adaptat ion , Neural Networks , 1 , 295–307, (1988).
[20] T. P. Vogl, J. K. Man gis, J.K. Rigler, W. T. Z ink and D .L. Alkon, Accelerating the convergence of the back-propagation method , Biological Cyberne tics, 59 , 257–263,(1988).
You do not have rights to view the full text article.
Please contact administration for subscription to Journal or individual article.
Mail us at support@isroset.org or view contact page for more details.