Full Paper View Go Back
Application of Fuzzy Expert system in Estimating LaborProductivity
Deepak Sharma1 , GarimaSolanki 2 , Anjali Upadhyay3
Section:Survey Paper, Product Type: Journal-Paper
Vol.7 ,
Issue.4 , pp.35-39, Dec-2020
Online published on Dec 31, 2020
Copyright © Deepak Sharma, GarimaSolanki, Anjali Upadhyay . This is an open access article distributed under the Creative Commons Attribution License, which permits unrestricted use, distribution, and reproduction in any medium, provided the original work is properly cited.
View this paper at Google Scholar | DPI Digital Library
How to Cite this Paper
- IEEE Citation
- MLA Citation
- APA Citation
- BibTex Citation
- RIS Citation
IEEE Style Citation: Deepak Sharma, GarimaSolanki, Anjali Upadhyay, “Application of Fuzzy Expert system in Estimating LaborProductivity,” World Academics Journal of Engineering Sciences, Vol.7, Issue.4, pp.35-39, 2020.
MLA Style Citation: Deepak Sharma, GarimaSolanki, Anjali Upadhyay "Application of Fuzzy Expert system in Estimating LaborProductivity." World Academics Journal of Engineering Sciences 7.4 (2020): 35-39.
APA Style Citation: Deepak Sharma, GarimaSolanki, Anjali Upadhyay, (2020). Application of Fuzzy Expert system in Estimating LaborProductivity. World Academics Journal of Engineering Sciences, 7(4), 35-39.
BibTex Style Citation:
@article{Sharma_2020,
author = {Deepak Sharma, GarimaSolanki, Anjali Upadhyay},
title = {Application of Fuzzy Expert system in Estimating LaborProductivity},
journal = {World Academics Journal of Engineering Sciences},
issue_date = {12 2020},
volume = {7},
Issue = {4},
month = {12},
year = {2020},
issn = {2347-2693},
pages = {35-39},
url = {https://www.isroset.org/journal/WAJES/full_paper_view.php?paper_id=2203},
publisher = {IJCSE, Indore, INDIA},
}
RIS Style Citation:
TY - JOUR
UR - https://www.isroset.org/journal/WAJES/full_paper_view.php?paper_id=2203
TI - Application of Fuzzy Expert system in Estimating LaborProductivity
T2 - World Academics Journal of Engineering Sciences
AU - Deepak Sharma, GarimaSolanki, Anjali Upadhyay
PY - 2020
DA - 2020/12/31
PB - IJCSE, Indore, INDIA
SP - 35-39
IS - 4
VL - 7
SN - 2347-2693
ER -
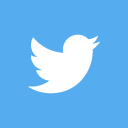
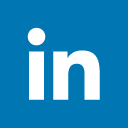
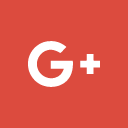
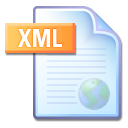
Abstract :
Fuzzy expert system have been utilized to tackle complex issues productively for the situation where data accessible is in spellbinding structure as opposed to quantitative number. This examination has intended to utilize Fuzzy expert system to assess the work creation rates through joining the impact of subjective and quantitative factor.
In this examination, to assess the Work Efficiency in any industry we have utilized wide information assortment, poll study and by talking industry personals. This task works fundamental expects to distinguish the variables influencing the Work Profitability in any industry. The 50 components of assessing Work Efficiency are browsed the modern tasks. The review was done through wide information assortment, poll overview and specialists sees which are then positioned through RII scale. The positioning is done through RII and afterward it is utilized for choosing highest level components which are answerable for Work Efficiency.
Key-Words / Index Term :
Labor Productivity, fuzzy logic, simulation, Prediction, decision-making, soft computing, MATLAB
References :
[1] Kapil Mittal &Puran Chandra Tewari& Dinesh Khanduja, (2017) “Productivity improvement under manufacturing environment using Shainin system and fuzzy analytical hierarchy process: a case study”, The International Journal of Advanced Manufacturing Technology, Volume 92, Issue 1–4, pp 407–421.
[2] Toly Chen, (2016) “New fuzzy method for improving the precision of productivity predictions for a factory”, Neural Computation & Application, Issue 11.
[3] Thomas Czumanski and Hermann Lödding, (2016) “State-based analysis of labor productivity”, International Journal of Production Research, Taylor and Francis, Volume 54, Issue 10.
[4] PouyaNojedehi&FarnadNasirzadeh, (2016) “A Hybrid Simulation Approach to Model and Improve Construction Labor Productivity”, KSCE Journal of Civil Engineering, Volume 21, Issue 5, pp 1516–1524.
[5] Laptal Oral, Mustafa Oral, Mehmet Anda, (2016) “Construction Crew Productivity Prediction: Application of Two Novel Methods”, Int. Journal Civil Engineering, pp 181–186.
[6] SusantiRachman, Mark A Gregory, Seema W Narayan, (2015) “The Role of ICT Services on Indonesian Small to Medium Enterprise Productivity”, International Telecommunication Networks, pp 166-172.
[7] SeungjunAhn, A. J. Antony Chettupuzha, Ronald Ekyalimpa, (2015) “Fuzzy Cognitive Maps as a Tool for Modeling Construction Labor Productivity” Annual Conference of the North American Fuzzy Information Processing Society (NAFIPS).
[8] Aminah Robinson Fayek, Abraham AssefaTsehayae, (2012) “Modeling Construction Labor Productivity Using Fuzzy Logic and Exploring the Use of Fuzzy Hybrid Techniques” Annual Meeting of the North American Fuzzy Information Processing Society (NAFIPS), IEEE, Berkeley, CA.
[9] MohdFarisKhamidi, Yap KeemSiah, Muhammad Saqib, “Application of Fuzzy Expert Systems for Construction Labor Productivity Estimation”, International Conference on Computer & Information Science (ICCIS), IEEE, Volume 2, pp 506-511.
[10] Gilberto A. Corona-Suárez, Simaan M. AbouRizk, and Stanislav Karapetrovic, (2014) “Simulation-Based Fuzzy Logic Approach to Assessing the Effect of Project Quality Management on Construction Performance”, Journal of Quality and Reliability Engineering, Hindawi Publishing Corporation, Volume 2014, Article ID 203427, vol. 2014, 18 pages.
[11] M. Arashpour, (2015) “Analysis of Workflow Variability and Its Impacts on Productivity and Performance in Construction of Multistory Buildings”, Journal of Management in Engineering, Vol. 31, Issue 6.
[12] TJ Coelli TJ, DSP Rao, CJ O’Donnell, GE Battese, (2005) “An introduction to efficiency and productivity analysis”, second edition,Springer, Berlin.
[13] K. El-Gohary, R. Aziz, (2014) “Factors influencing construction labor productivity in Egypt”, Journal of Management in Engineering, Vol. 30, No. 1, pp. 1-9.
[14] YC Wang, T Chen, (2013) “A fuzzy collaborative forecasting approach for forecasting the productivity of a factory”, Adv Mechanical Engineering 5: page 1–13.
[15] F. Nasirzadeh, A. Afshar, M. Khanzadi, and S. Howick, (2008) “Integrating system dynamics and fuzzy logic modeling for construction risk management”, Construction Management and Economics, Vol. 26, issue 11, pp. 1197-1212.
[16] Ars hinder, A Kanda, SG Deshmukh, (2007) “Coordination in supply chains: an evaluation using fuzzy logic”, Production Plan Control 18: Volume 18, Issue 5, pp. 420– 435.
[17] J Beg, MS Shunmugam, (2003) “Application of fuzzy logic in the selection of part orientation and probe orientation sequencing for prismatic parts” International Journal Prod Res 41, Volume 41, Issue 12, pp.2799–2815.
[18] S Nandy, PP Sarkar, A Das, (2012) “Training a feed-forward neural network with artificial bee colony-based backpropagation method”, International Journal of Computer Science & Information Technology (IJCSIT), Vol 4, No 4, 33-46
[19] H. R. Thomas, and R. D. Ellis, (2009) “Fundamental Principles of Weather Mitigation.” Practice Periodical on Structural Design and Construction, Vol. 14, No. 1, pp 29-35.
[20] M Oral, E Oral, (2007) “A computer-based system for documentation and monitoring of construction labor productivity”, CIB 24th W78 conference, Maribor.
[21] AK Sangaiah, PR Subramaniam, X Zheng, (2015) “A combined fuzzy DEMATEL and fuzzy TOPSIS approach for evaluating GSD project outcome factors”, Neural Computing and Applications, Volume 26, Issue 5, pp 1025–1040.
[22] T Chen, (2015) “A fuzzy back-propagation network approach for planning actions to shorten the cycle time of a job in dynamic random-access memory manufacturing”, Neural Computing and Applications: Volume 26, Issue 8, pp 1813–1825.
[23] G Peters, (1994) “Fuzzy linear regression with fuzzy intervals”, Fuzzy Sets Syst 63: pp 45–55.
[24] Chen T, (2007) “Evaluating the mid-term competitiveness of a product in a semiconductor fabrication factory with a systematic procedure”, Computational Ind Engineering 53: pp. 499–513.
[25] Dissanayake M, Fayek AR, Russell AD, Pedrycz W, (2005) “A hybrid neural network for predicting construction labour productivity”, Computational Civil Engineering ASCE, PP. 303–328.
[26] Di Fana, Chris K.Y. Lob, Andy C.L. Yeungc, T.C.E. Chengc, (2018) “The impact of corporate label change on long-term labor productivity”, Journal of Business Research 86, pp 96–108.
[27] Michele Battisti, Massimo Del Gatto, Christopher F. Parmeter, (2017) “Labor productivity growth: disentangling technology and capital accumulation”, Journal of Economic Growth, Springer, Volume 23, Issue 1, pp 111–143.
[28] Dave R. Bonham, Paul M. Goodrum, Ray Littlejohn, Mohammed A. Albertha, (2017) “Application of Data Mining Techniques to Quantify the Relative Influence of Design and Installation Characteristics on Labor Productivity”, Journal of Construction Engineering Management, American Society of Civil Engineers, Volume 143, Issue 8.
[29] Mario Coccia, (2017) “Optimization in R&D intensity and tax on corporate profits for supporting labor productivity of nations”, The Journal of Technology Transfer, Springer, Volume 43, Issue 3, pp 792–814.
[30] Nirajan Mani, Krishna P. Kisi, Eddy M. Rojas and E. Terence Foster, (2017) “Estimating Construction Labor Productivity Frontier: Pilot Study”, Journal of Construction Engineering Management, American Society of Civil Engineers, Volume 143, Issue 10.
[31] Joanna Tyrowicz, Lucas van der Velde, Jan Svejnar, (2016) “Effects of Labor Reallocation on Productivity and Inequality—Insights from Studies on Transition”, Journal of Economic Surveys, John Wiley & Sons Ltd, Vol. 00, No. 0, pp. 1–21.
[32] Christopher F. Baum, Hans Lööf, PardisNabavi& Andreas Stephan, (2016) “A new approach to estimation of the R&D–innovation productivity relationship”, Economics of Innovation and New Technology, Volume 26, Issue 1-2, Pages 121-133.
[33] Erik A. Poirier, Sheryl Staub-French, Daniel Forgues, (2015) “Measuring the impact of BIM on labor productivity in a small specialty contracting enterprise through action-research”, Automation in Construction 58, Elsevier, Volume 58, Pages 74-84.
[34] GholamrezaHeravi and Ehsan Eslamdoost, (2015) “Applying Artificial Neural Networks for Measuring and Predicting Construction-Labor Productivity”, Journal Construction Eng. Management, American Society of Civil Engineers, Volume 141 Issue 10.
[35] Rohit B. Ganorkar and K. N. Agrawal, (2018) “Process Optimization in Continuous Corrugation Line at Steel Processing Industry”, Industrial Engineering Journal, Volume 10, Issue 7, pp 27-31.
[36] Divyesh Prakash Trivedi, B. B Ahu and N. R. Rajhans, (2018) “Process Optimization of Plastic Tap Manufacturing Company”, Industrial Engineering Journal, Volume 10 (10).
[37] Ravi Nagaich Shukla, Apratul Chandra and Rishabh Mishra, (2018) “Productivity Improvement by Using Method Study in Automobile Industry: A Case Study”, Industrial Engineering Journal, Volume 10 (9).
You do not have rights to view the full text article.
Please contact administration for subscription to Journal or individual article.
Mail us at support@isroset.org or view contact page for more details.