Full Paper View Go Back
Online Intrusion Alert Aggregation with Generative Data Stream Modeling
Ramchandar Durgam1 , R.V.Krishnaiah 2
Section:Technical Paper, Product Type: Isroset-Journal
Vol.1 ,
Issue.5 , pp.23-23, Sep-2013
Online published on Oct 30, 2013
Copyright © Ramchandar Durgam , R.V.Krishnaiah . This is an open access article distributed under the Creative Commons Attribution License, which permits unrestricted use, distribution, and reproduction in any medium, provided the original work is properly cited.
View this paper at Google Scholar | DPI Digital Library
How to Cite this Paper
- IEEE Citation
- MLA Citation
- APA Citation
- BibTex Citation
- RIS Citation
IEEE Style Citation: Ramchandar Durgam , R.V.Krishnaiah, “Online Intrusion Alert Aggregation with Generative Data Stream Modeling,” International Journal of Scientific Research in Computer Science and Engineering, Vol.1, Issue.5, pp.23-23, 2013.
MLA Style Citation: Ramchandar Durgam , R.V.Krishnaiah "Online Intrusion Alert Aggregation with Generative Data Stream Modeling." International Journal of Scientific Research in Computer Science and Engineering 1.5 (2013): 23-23.
APA Style Citation: Ramchandar Durgam , R.V.Krishnaiah, (2013). Online Intrusion Alert Aggregation with Generative Data Stream Modeling. International Journal of Scientific Research in Computer Science and Engineering, 1(5), 23-23.
BibTex Style Citation:
@article{Durgam_2013,
author = {Ramchandar Durgam , R.V.Krishnaiah},
title = {Online Intrusion Alert Aggregation with Generative Data Stream Modeling},
journal = {International Journal of Scientific Research in Computer Science and Engineering},
issue_date = {9 2013},
volume = {1},
Issue = {5},
month = {9},
year = {2013},
issn = {2347-2693},
pages = {23-23},
url = {https://www.isroset.org/journal/IJSRCSE/full_paper_view.php?paper_id=89},
publisher = {IJCSE, Indore, INDIA},
}
RIS Style Citation:
TY - JOUR
UR - https://www.isroset.org/journal/IJSRCSE/full_paper_view.php?paper_id=89
TI - Online Intrusion Alert Aggregation with Generative Data Stream Modeling
T2 - International Journal of Scientific Research in Computer Science and Engineering
AU - Ramchandar Durgam , R.V.Krishnaiah
PY - 2013
DA - 2013/10/30
PB - IJCSE, Indore, INDIA
SP - 23-23
IS - 5
VL - 1
SN - 2347-2693
ER -
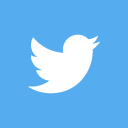
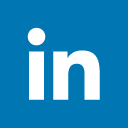
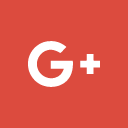
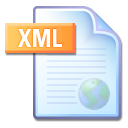
Abstract :
Security plays an important role in IT systems. Intrusion detection systems can be used to ensure security in a network. The existing IDSs (Intrusion Detection Systems) such as Firewall, Snort provide huge number of alerts as they monitor the network flows. Since the number of alerts is plenty, the network administrator might be confused to know exact problem. This will delay indecision making in the presence of any security threats. As it takes more time to understand the alerts when they are more number, the network administrator needs to spend some time to make effective decisions. In this paper, we proposed a framework which aggregates alerts and generates few Meta alerts. These Meta alerts can be understood by the network personnel quickly and take decisions immediately. A data stream version of maximum likelihood approach is used in the framework. The experimental results revealed that the framework is very useful and can be used in the real world networks.
Key-Words / Index Term :
IDS, Online Intrusion Detection, Probabilistic Model, Online Intrusion Detection, Alert Aggregation
References :
[1] S. Axelsson, ―Intrusion Detection Systems: A Survey and Taxonomy,‖ Technical Report 99-15, Dept. of Computer Eng., Chalmers Univ. Of Technology, 2000.
[2] C.M. Bishop, Pattern Recognition and Machine Learning. Springer, 2006.
[3] M.R. Henzinger, P. Raghavan, and S. Rajagopalan, Computing on Data Streams. Am. Math. Soc., 1999.
[4] A. Allen, ―Intrusion Detection Systems: Perspective,‖ Technical Report DPRO-95367, Gartner, Inc., 2003.
[5] F. Valeur, G. Vigna, C. Krugel, and R.A. Kemmerer, ―A Comprehensive Approach to Intrusion Detection Alert Correla- tion,‖ IEEE Trans. Dependable and Secure Computing, vol. 1, no. 3, pp. 146-169, July-Sept. 2004.
[6] H. Debar and A. Wespi, ―Aggregation and Correlation of Intrusion-Detection Alerts,‖ Recent Advances in Intrusion Detection, W. Lee, L. Me, and A. Wespi, eds., pp. 85-103, Springer, 2001.
[7] D. Li, Z. Li, and J. Ma, ―Processing Intrusion Detection Alerts in Large-Scale Network,‖ Proc. Int’l Symp. Electronic Commerce and Security, pp. 545-548, 2008.
[8] F. Cuppens, ―Managing Alerts in a Multi-Intrusion Detection Environment,‖ Proc. 17th Ann. Computer Security Applications Conf. (ACSAC ’01), pp. 22-31, 2001.
[9] A. Valdes and K. Skinner, ―Probabilistic Alert Correlation,‖ Recent Advances in Intrusion Detection, W. Lee, L. Me, and A. Wespi, eds. pp. 54-68, Springer, 2001.
[10] K. Julisch, ―Using Root Cause Analysis to Handle IntrusionDetection Alarms,‖ PhD dissertation, Universitat
Dortmund, 2003. 294
[12] T. Pietraszek, ―Alert Classification to Reduce False Positives in ̈Intrusion Detection ,‖ PhD dissertation, Universitat Freiburg, 2006.
[13] F. Autrel and F. Cuppens, ―Using an Intrusion Detection Alert Similarity Operator to Aggregate and Fuse Alerts,‖
Proc. Fourth Conf. Security and Network Architectures, pp. 312-322, 2005.
[14] G. Giacinto, R. Perdisci, and F. Roli, ―Alarm Clustering for Intrusion Detection Systems in Computer Networks,‖ Machine Learning and Data Mining in Pattern Recognition, P. Perner and A. Imiya, eds. pp. 184-193, Springer, 2005.
[15] O. Dain and R. Cunningham, ―Fusing a Heterogeneous Alert Stream into Scenarios,‖ Proc. 2001 ACM Workshop
Data Mining for Security Applications, pp. 1-13, 2001.
[16] P. Ning, Y. Cui, D.S. Reeves, and D. Xu, ―Techniques and Tools for Analyzing Intrusion Alerts,‖ ACM Trans. Information Systems Security, vol. 7, no. 2, pp. 274-318, 2004.
[17] F. Cuppens and R. Ortalo, ―LAMBDA: A Language to Model a Database for Detection of Attacks,‖ Recent Advances in Intrusion Detection, H. Debar, L. Me, and S.F. Wu, eds. pp. 197-216, Springer, 2000.
[18] S.T. Eckmann, G. Vigna, and R.A. Kemmerer, ―STATL: An Attack Language for State-Based Intrusion Detection,‖ J.
Computer Security, vol. 10, nos. 1/2, pp. 71-103, 2002.
[19] A. Hofmann, ―Alarmaggregation und Interessantheitsbewertung in einem dezentralisierten Angriffserkennungsystem,‖ PhD dis- ̈sertation, Universitat Passau, under review.
[20] M.S. Shin, H. Moon, K.H. Ryu, K. Kim, and J. Kim, ―Applying Data Mining Techniques to Analyze Alert Data,‖ Web Technologies and Applications, X. Zhou, Y. Zhang, and M.E. Orlowska, eds. pp. 193-200, Springer, 2003.
[21] J. Song, H. Ohba, H. Takakura, Y. Okabe, K. Ohira, and Y. Kwon, ―A Comprehensive Approach to Detect Unknown Attacks via Intrusion Detection Alerts,‖ Advances in Computer Science—ASIAN 2007, Computer and Network Security, I. Cervesato, ed., pp. 247-253, Springer, 2008.
[22] R. Smith, N. Japkowicz, M. Dondo, and P. Mason, ―Using Unsupervised Learning for Network Alert Correlation,‖ Advances in Artificial Intelligence, R. Goebel, J. Siekmann, and W. Wahlster, eds. pp. 308-319, Springer, 2008.
You do not have rights to view the full text article.
Please contact administration for subscription to Journal or individual article.
Mail us at support@isroset.org or view contact page for more details.