Full Paper View Go Back
Unleashing the Power of AI and Machine Learning: Integration Strategies for IoT Systems
S. Mohanarangan1 , D. Karthika2 , B. Moohambigai3 , R. Sangeetha4
Section:Research Paper, Product Type: Journal-Paper
Vol.12 ,
Issue.2 , pp.25-32, Apr-2024
Online published on Apr 30, 2024
Copyright © S. Mohanarangan, D. Karthika, B. Moohambigai, R. Sangeetha . This is an open access article distributed under the Creative Commons Attribution License, which permits unrestricted use, distribution, and reproduction in any medium, provided the original work is properly cited.
View this paper at Google Scholar | DPI Digital Library
How to Cite this Paper
- IEEE Citation
- MLA Citation
- APA Citation
- BibTex Citation
- RIS Citation
IEEE Style Citation: S. Mohanarangan, D. Karthika, B. Moohambigai, R. Sangeetha, “Unleashing the Power of AI and Machine Learning: Integration Strategies for IoT Systems,” International Journal of Scientific Research in Computer Science and Engineering, Vol.12, Issue.2, pp.25-32, 2024.
MLA Style Citation: S. Mohanarangan, D. Karthika, B. Moohambigai, R. Sangeetha "Unleashing the Power of AI and Machine Learning: Integration Strategies for IoT Systems." International Journal of Scientific Research in Computer Science and Engineering 12.2 (2024): 25-32.
APA Style Citation: S. Mohanarangan, D. Karthika, B. Moohambigai, R. Sangeetha, (2024). Unleashing the Power of AI and Machine Learning: Integration Strategies for IoT Systems. International Journal of Scientific Research in Computer Science and Engineering, 12(2), 25-32.
BibTex Style Citation:
@article{Mohanarangan_2024,
author = {S. Mohanarangan, D. Karthika, B. Moohambigai, R. Sangeetha},
title = {Unleashing the Power of AI and Machine Learning: Integration Strategies for IoT Systems},
journal = {International Journal of Scientific Research in Computer Science and Engineering},
issue_date = {4 2024},
volume = {12},
Issue = {2},
month = {4},
year = {2024},
issn = {2347-2693},
pages = {25-32},
url = {https://www.isroset.org/journal/IJSRCSE/full_paper_view.php?paper_id=3461},
publisher = {IJCSE, Indore, INDIA},
}
RIS Style Citation:
TY - JOUR
UR - https://www.isroset.org/journal/IJSRCSE/full_paper_view.php?paper_id=3461
TI - Unleashing the Power of AI and Machine Learning: Integration Strategies for IoT Systems
T2 - International Journal of Scientific Research in Computer Science and Engineering
AU - S. Mohanarangan, D. Karthika, B. Moohambigai, R. Sangeetha
PY - 2024
DA - 2024/04/30
PB - IJCSE, Indore, INDIA
SP - 25-32
IS - 2
VL - 12
SN - 2347-2693
ER -
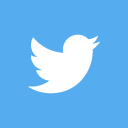
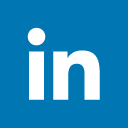
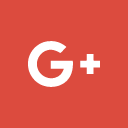
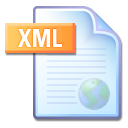
Abstract :
In the realm of the Internet of Things (IoT), the integration of Artificial Intelligence (AI) and Machine Learning (ML) stands as a transformative force, offering unparalleled capabilities to leverage the vast amounts of data generated by connected devices. This abstract delves into the strategies and implications of integrating AI and ML within IoT systems, elucidating key concepts, challenges, and opportunities. The amalgamation of AI and ML with IoT enables enhanced data analysis, predictive insights, and autonomous decision-making at the edge of the network. This synergy empowers IoT devices to not only collect data but also interpret it intelligently, paving the way for predictive maintenance, anomaly detection, and optimization of operational processes. Keywords such as "edge computing," "real-time analytics," and "predictive maintenance" underscore the pivotal role of AI and ML in maximizing the efficiency and efficacy of IoT deployments. One of the primary challenges in this integration lies in the efficient processing and analysis of data amidst the constraints of IoT devices, including limited computational power and bandwidth. Edge computing emerges as a solution, facilitating data processing closer to the data source, thereby reducing latency and conserving network resources. Additionally, the utilization of lightweight ML algorithms optimized for resource-constrained environments becomes imperative, ensuring the feasibility of AI-powered applications on IoT devices. Furthermore, the integration of AI and ML within IoT extends beyond traditional use cases, permeating diverse domains such as healthcare, manufacturing, transportation, and smart cities. In healthcare, IoT-enabled wearables and medical devices coupled with AI-driven analytics revolutionize patient care, enabling remote monitoring, early disease detection, and personalized treatment. Similarly, in manufacturing, Industrial IoT (IIoT) solutions empowered by AI and ML optimize production processes, enhance quality control, and enable predictive maintenance, thereby augmenting productivity and competitiveness.
Key-Words / Index Term :
IoT, Artificial Intelligence, Machine Learning, Edge Computing, Predictive Maintenance, Cybersecurity, Interoperability Standards, Healthcare, Industrial IoT (IIoT), Smart Cities.
References :
[1] Suyog Gupta, Ankur Agrawal, Kailash Gopalakrishnan, Pritish Narayanan, Deep Learning with Limited Numerical Precision, International Conference on Machine Learning (ICML), pp 1737-1746, 2015.
[2] Han, Song, Mao, Huizi, Dally, William J., Deep Compression: Compressing Deep Neural Networks with Pruning, Trained Quantization and Huffman Coding, International Conference on Learning Representations (ICLR), 2016.
[3] Wen, Wei, Wu, Chunpeng, Wang, Yandan, Chen, Yiran, Li, Hai, Learning Structured Sparsity in Deep Neural Networks, Advances in Neural Information Processing Systems (NeurIPS), pp 2074-2082, 2016.
[4] D`Agostino, Daniele, Prete, Cosimo A., Zavagli, Francesco, Real-time filtering of IoT data streams based on MQTT and Spark Streaming, IEEE International Conference on Industrial Technology (ICIT), pp 1184-1189, 2016.
[5] Renganarayana, Suresh, Vasilakos, Athanasios V., Bhatnagar, Sushil, The era of edge computing Journal: IEEE Consumer Electronics Magazine, pp 60-67, 2017
[6] Xu, Chao, Ren, Jie, Yin, Jian, Jin, Tian, Energy-efficient resource allocation for device-to-device communication underlaying cellular networks, IEEE Transactions on Wireless Communications, pp 1546-1559, 2016.
[7] Chen, Li, Xie, Xinghua, Cheng, Bo, Hu, Xiao, Yang, Jian, Energy-Efficient Resource Allocation for Network-Coding-Based Device-to-Device Communications, IEEE Transactions on Vehicular Technology, pp 1917-1927, 2018.
[8] Tang, Tao, Liao, Jianxin, Xiong, Guanglin, Jiang, Tao, Liu, Sheng, Energy Efficient Resource Allocation for Network MIMO Systems with SWIPT, IEEE Transactions on Vehicular Technology, pp 3731-3742, 2018.
[9] Zhang, Kai, Wang, Wei, Liu, Wei, Energy-Efficient Resource Allocation for Full-Duplex Multicast Relay Networks, IEEE Transactions on Communications, pp 3044-3058, 2017.
[10] Nasir, Hafeez Ur Rehman, Zhou, Qing, Energy-efficient resource allocation in network-controlled device-to-device communications, IET Communications, pp 867-874, 2017.
[11] Yang, Zhijie, Zhou, Xing, Chen, Qing, Wang, Ning, Zou, Zhimin, On the Feasibility of AI-Driven IoT Through Low-Resolution Event Logging, IEEE Internet of Things Journal, pp 69-82, 2019.
[12] Kim, Beomseok, Park, Soojeong, Chung, Kiseon, Feasibility of Artificial Intelligence and Machine Learning Techniques for IoT Devices, Journal of Internet Technology, pp 1637-1646, 2019.
[13] Liu, Zhiyuan, Guo, Mengmeng, Qi, Li, Shen, Xiaohong, Li, Shancang, Feasibility Analysis of Applying Deep Learning in IoT Security, IEEE Access, pp 12695-12703, 2019.
[14] Kang, Minwoo, Kang, Sehun, Kim, Taeho, Lee, Juho, Feasibility Study on IoT Devices for Deep Learning Model Training, Proceedings of the 2019 ACM International Joint Conference on Pervasive and Ubiquitous Computing and Proceedings of the 2019 ACM International Symposium on Wearable Computers, pp 505-508, 2019.
[15] Liu, Qingxin, Li, Yongxiang, Ma, Jiayang, Zhang, Xi, Zhou, Wen, Feasibility study of deploying deep learning models on wearable devices for daily activity recognition, Journal of Ambient Intelligence and Humanized Computing, 2021.
[16] Poorana Senthilkumar S, Subramani B, "RPL Protocol Load balancing Schemes in Low-Power and Lossy Networks", International Journal of Scientific Research in Computer Science and Engineering, Vol.11, Issue.1, pp.07-13, February 2023.
[17] Vidhi Tiwari, Pratibha Adkar, "Implementation of IoT in Home Automation using android application", Vol.7, Issue.2, pp.11-16, April 2019, DOI: https://doi.org/10.26438/ijsrcse/v7i2.1116.
You do not have rights to view the full text article.
Please contact administration for subscription to Journal or individual article.
Mail us at support@isroset.org or view contact page for more details.