Full Paper View Go Back
An Attribute-Assisted Reranking Model for Web Image Search
ST. Tangudubilli1 , AS. Kumar2
Section:Review Paper, Product Type: Isroset-Journal
Vol.4 ,
Issue.3 , pp.20-25, Jun-2016
Online published on Jul 02, 2016
Copyright © ST. Tangudubilli, AS. Kumar . This is an open access article distributed under the Creative Commons Attribution License, which permits unrestricted use, distribution, and reproduction in any medium, provided the original work is properly cited.
View this paper at Google Scholar | DPI Digital Library
How to Cite this Paper
- IEEE Citation
- MLA Citation
- APA Citation
- BibTex Citation
- RIS Citation
IEEE Style Citation: ST. Tangudubilli, AS. Kumar, “An Attribute-Assisted Reranking Model for Web Image Search,” International Journal of Scientific Research in Computer Science and Engineering, Vol.4, Issue.3, pp.20-25, 2016.
MLA Style Citation: ST. Tangudubilli, AS. Kumar "An Attribute-Assisted Reranking Model for Web Image Search." International Journal of Scientific Research in Computer Science and Engineering 4.3 (2016): 20-25.
APA Style Citation: ST. Tangudubilli, AS. Kumar, (2016). An Attribute-Assisted Reranking Model for Web Image Search. International Journal of Scientific Research in Computer Science and Engineering, 4(3), 20-25.
BibTex Style Citation:
@article{Tangudubilli_2016,
author = {ST. Tangudubilli, AS. Kumar},
title = {An Attribute-Assisted Reranking Model for Web Image Search},
journal = {International Journal of Scientific Research in Computer Science and Engineering},
issue_date = {6 2016},
volume = {4},
Issue = {3},
month = {6},
year = {2016},
issn = {2347-2693},
pages = {20-25},
url = {https://www.isroset.org/journal/IJSRCSE/full_paper_view.php?paper_id=278},
publisher = {IJCSE, Indore, INDIA},
}
RIS Style Citation:
TY - JOUR
UR - https://www.isroset.org/journal/IJSRCSE/full_paper_view.php?paper_id=278
TI - An Attribute-Assisted Reranking Model for Web Image Search
T2 - International Journal of Scientific Research in Computer Science and Engineering
AU - ST. Tangudubilli, AS. Kumar
PY - 2016
DA - 2016/07/02
PB - IJCSE, Indore, INDIA
SP - 20-25
IS - 3
VL - 4
SN - 2347-2693
ER -
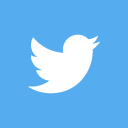
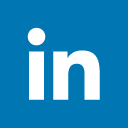
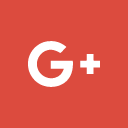
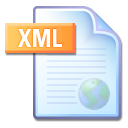
Abstract :
Image search reranking is a successful approach to refine the text-based image search result. Most existing reranking ways are based on low level visual features. This paper proposes to make use of semantic attributes for image search reranking. Depend on the classifiers for all the predefined attributes, each image is represented by an attribute feature containing the responses from these classifiers. A hypergraph is then used to model the relationship between images by combining low level visual features and attribute features. Hypergraph ranking is then performed to order the images. The basic principle is that visually similar images should have similar ranking scores. In this paper, we propose a visual attribute joint hypergraph learning approach at the same time to explore two information sources. A hypergraph is created to model the relationship of all images. We conduct experiments on more than 1,000 queries in MSRA-MMV2.0 data set. The experimental results indicate the productiveness of our approach.
Key-Words / Index Term :
Text base query; Attribute-assisted; Image retrieval; Query image; hyper graph learning; Image reranking
References :
[1]. J. Cai, ZJ Zha, M. Wang, S. Zhang, Q. Tian, “An Attribute-Assisted Reranking Model for Web Image Search”, IEEE Transactions On Image Processing, Vol. 24, No. 1, pp.261-272, 2015.
[2]. L. Yang, A. Hanjalic, “Prototype-Based Image Search Reranking”, IEEE Transactions On Multimed, Vol.14, No.3,pp. 871-88, 2012.
[3]. S. Changpinyo, E. Sudderth, “Learning image attributes using the Indian Buffet Process”, Technical report ofBrown University, USA, pp.1-146, 2012.
[4]. B. Siddiquie, R.S. Feris, L.S. Davis, “Image ranking and retrieval based on multi-attribute queries”, IEEE Conference on Computer Vision and Pattern Recognition (CVPR), Providence, pp.801-808, 2011 .
[5]. J. Feng, S. Jegelka, S. Yan and T. Darrell, "Learning Scalable Discriminative Dictionary with Sample Relatedness", 2014 IEEE Conference on Computer Vision and Pattern Recognition, Columbus, pp.1645-1652, 2014.
[6]. N. Kumar, A. C. Berg, P. N. Belhumeur and S. K. Nayar, "Attribute and simile classifiers for face verification," 2009 IEEE 12th International Conference on Computer Vision, Kyoto, pp.365-372, 2009.
[7]. M. Wang, L. Yang, X.-S.Hua, “MSRA-MM: Bridging research and industrial societies for multimedia,” Tech. Rep. MSR-TR-2009-30, 2009.
[8]. K. Järvelin, J. Kekäläinen, “IR evaluation methods for retrieving highly relevant documents”, 23rd annual international ACM SIGIR conference on Research and development in information retrieval, Greece , pp.41–48, 2000.
[9]. W. H. Hsu, L. S. Kennedy, S.-F.Chang, “Video search reranking via information bottleneck principle”, Proceedings of the 14th ACM international conference on Multimedia, Santa Barbara, pp.35–44, 2006.
[10]. Y. Huang, Q. Liu, S. Zhang, D.N. Metaxas, “Image retrieval via probabilistic hypergraph ranking”, IEEE Computer Society Conference on Computer Vision and Pattern Recognition, San Francisco, pp.3376–3383, 2010.
[11]. C. H. Lampert, H. Nickisch, S. Harmeling, “Learning to detect unseen object classes by betweenclass attribute transfer”, IEEE Conference on Computer Vision and Pattern Recognition, Miami, pp. 951–958, 2009.
[12]. R. Yan, A. Hauptmann, and R. Jin, “Multimedia search with pseudo- relevance feedback”, Proceedings of the 2nd international conference on Image and video retrieval, Urbana-Champaign, pp. 238–247, 2003.
You do not have rights to view the full text article.
Please contact administration for subscription to Journal or individual article.
Mail us at support@isroset.org or view contact page for more details.